Got it! Big thanks to @jdhao for this answer. (C'mon, check it out and upvote!)
Here's the code for the source data again - I've added some more data to improve the example:
Id | PROC_NAME | START_TS | END_TS
---------------------------------------------------------------------
0 | data_load | 2019-06-25 03:30:00 | 2019-06-25 03:51:00
1 | data_send | 2019-06-25 07:15:00 | 2019-06-25 07:52:00
2 | data_load | 2019-06-26 03:30:00 | 2019-06-26 03:40:00
3 | data_send | 2019-06-26 07:19:00 | 2019-06-26 07:43:00
4 | data_load | 2019-06-26 08:54:00 | 2019-06-26 09:21:00
5 | data_send | 2019-06-27 03:30:00 | 2019-06-27 04:16:00
6 | data_load | 2019-06-27 08:51:00 | 2019-06-27 09:32:00
7 | data_send | 2019-06-28 03:30:00 | 2019-06-28 04:02:00
8 | data_extraction | 2019-06-25 03:21:00 | 2019-06-25 03:51:00
9 | data_extraction | 2019-06-25 06:45:00 | 2019-06-25 07:32:00
10 | data_extraction | 2019-06-26 03:30:00 | 2019-06-26 06:40:00
11 | data_extraction | 2019-06-26 07:19:00 | 2019-06-26 07:43:00
12 | data_extraction | 2019-06-26 10:54:00 | 2019-06-26 11:21:00
13 | data_extraction | 2019-06-27 05:30:00 | 2019-06-27 08:16:00
14 | data_extraction | 2019-06-27 09:51:00 | 2019-06-27 11:32:00
15 | data_extraction | 2019-06-28 02:30:00 | 2019-06-28 04:02:00
Here's the code for Jupyter:
import pandas as pd
import matplotlib.pyplot as plt
import matplotlib.dates as dt
df = pd.DataFrame(
{
'PROC_NAME': ['data_load', 'data_send', 'data_load', 'data_send', 'data_load', 'data_send', 'data_load', 'data_send',
'data_extraction', 'data_extraction', 'data_extraction', 'data_extraction', 'data_extraction', 'data_extraction', 'data_extraction', 'data_extraction',],
'START_TS': ['2019-06-25 03:30', '2019-06-25 07:15', '2019-06-26 03:30', '2019-06-26 07:19',
'2019-06-26 08:54', '2019-06-27 03:30', '2019-06-27 08:51', '2019-06-28 03:30',
'2019-06-25 03:21', '2019-06-25 06:45', '2019-06-26 03:30', '2019-06-26 07:19',
'2019-06-26 10:54', '2019-06-27 05:30', '2019-06-27 09:51', '2019-06-28 02:30'],
'END_TS': ['2019-06-25 03:51', '2019-06-25 07:52', '2019-06-26 03:40', '2019-06-26 07:43',
'2019-06-26 09:21', '2019-06-27 04:16', '2019-06-27 09:32', '2019-06-28 04:02',
'2019-06-25 03:51', '2019-06-25 07:32', '2019-06-26 06:40', '2019-06-26 07:43',
'2019-06-26 11:21', '2019-06-27 08:16', '2019-06-27 11:32', '2019-06-28 04:02']
})
#convert input to datetime:
df.START_TS = pd.to_datetime(df.START_TS, format = '%Y-%m-%d %H:%M')
df.END_TS = pd.to_datetime(df.END_TS, format = '%Y-%m-%d %H:%M')
df.head()
And the solution to my problem, using pyplot.hlines
:
fig = plt.figure()
fig.set_figheight(2)
fig.set_figwidth(15)
ax = fig.add_subplot(211)
plt.xticks(rotation='25')
#format dates on x axis
ax.xaxis.set_major_formatter(mdates.DateFormatter('%b %d %H:%M'))
ax = ax.xaxis_date()
ax = plt.hlines(df.PROC_NAME,
dt.date2num(df.START_TS),
dt.date2num(df.END_TS),
lw = 10, # make the lines wider and looking more like ribbon
color = 'b' # add some color
)
Finally, the result, where I'm able to clearly see run times and overlaps:
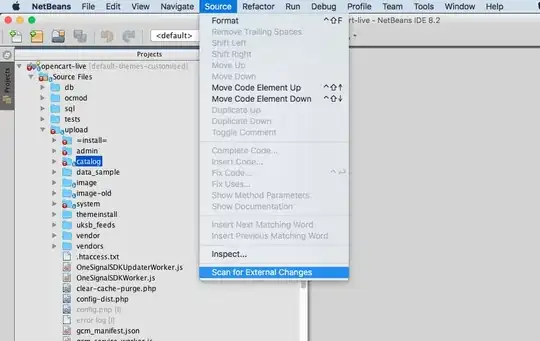