Convert your time series data into a numeric format with matplotlib.dats.date2num. Lay down a rectangular grid that spans your x and y ranges and do your convolution on that plot. Make a pseudo-color plot of your convolution and then reformat the x labels to be dates.
The label formatting is a little messy, but reasonably well documented. You just need to replace AutoDateFormatter with DateFormatter and an appropriate formatting string.
You'll need to tweak the constants in the convolution for your data.
import numpy as np
import datetime as dt
import pylab as plt
import matplotlib.dates as dates
t0 = dt.date.today()
t1 = t0+dt.timedelta(days=10)
times = np.linspace(dates.date2num(t0), dates.date2num(t1), 10)
dt = times[-1]-times[0]
price = 100 - (times-times.mean())**2
dp = price.max() - price.min()
volume = np.linspace(1, 100, 10)
tgrid = np.linspace(times.min(), times.max(), 100)
pgrid = np.linspace(70, 110, 100)
tgrid, pgrid = np.meshgrid(tgrid, pgrid)
heat = np.zeros_like(tgrid)
for t,p,v in zip(times, price, volume):
delt = (t-tgrid)**2
delp = (p-pgrid)**2
heat += v/( delt + delp*1.e-2 + 5.e-1 )**2
fig = plt.figure()
ax = fig.add_subplot(111)
ax.pcolormesh(tgrid, pgrid, heat, cmap='gist_heat_r')
plt.scatter(times, price, volume, marker='x')
locator = dates.DayLocator()
ax.xaxis.set_major_locator(locator)
ax.xaxis.set_major_formatter(dates.AutoDateFormatter(locator))
fig.autofmt_xdate()
plt.show()
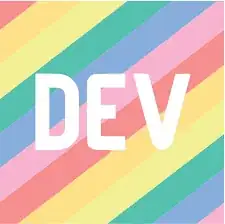