Don't let the name confuse you. The layer tf.keras.layers.Conv1D
needs the following shape: (time_steps, features)
. If your dataset is made of 10,000
samples with each sample having 64
values, then your data has the shape (10000, 64)
, which is not directly applicable to the tf.keras.layers.Conv1D
layer. You are missing the time_steps
dimension. What you can do is use the tf.keras.layers.RepeatVector
, which repeats your array input n
times, in the example 5
. This way your Conv1D
layer gets an input of the shape (5, 64)
. Check out the documentation for more information:
time_steps = 5
model = tf.keras.Sequential()
model.add(tf.keras.layers.Input(shape=(64,), name="input"))
model.add(tf.keras.layers.RepeatVector(time_steps))
model.add(tf.keras.layers.Conv1D(64, 2, activation="relu", padding="same", name="convLayer"))
As a side note, you should ask yourself if using a tf.keras.layers.Conv1D
layer is the right option for your use case. This layer is usually used for NLP and other time series tasks. For example, in sentence classification, each word in a sentence is usually mapped to a high-dimensional word vector representation, as seen in the image. This results in data with the shape (time_steps, features)
.
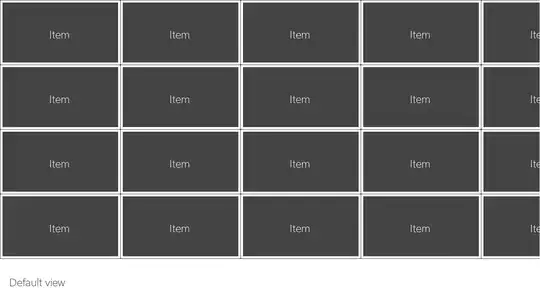
If you want to use character one hot encoded embeddings it would look something like this:
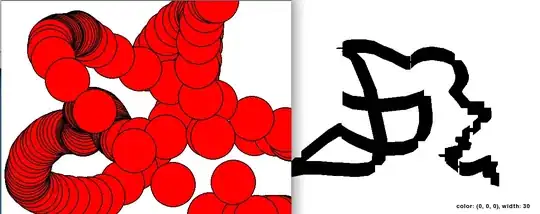
This is a simple example of one single sample with the shape (10, 10)
--> 10 characters along the time series dimension and 10 features. It should help you understand the tutorial I mentioned a bit better.