Currently, contrary to what has been stated in documentation, the Dense
layer is applied on the last axis of input tensor:
Contrary to the documentation, we don't actually flatten it. It's
applied on the last axis independently.
In other words, if a Dense
layer with m
units is applied on an input tensor of shape (n_dim1, n_dim2, ..., n_dimk)
it would have an output shape of (n_dim1, n_dim2, ..., m)
.
As a side note: this makes TimeDistributed(Dense(...))
and Dense(...)
equivalent to each other.
Another side note: be aware that this has the effect of shared weights. For example, consider this toy network:
model = Sequential()
model.add(Dense(10, input_shape=(20, 5)))
model.summary()
The model summary:
_________________________________________________________________
Layer (type) Output Shape Param #
=================================================================
dense_1 (Dense) (None, 20, 10) 60
=================================================================
Total params: 60
Trainable params: 60
Non-trainable params: 0
_________________________________________________________________
As you can see the Dense
layer has only 60 parameters. How? Each unit in the Dense
layer is connected to the 5 elements of each row in the input with the same weights, therefore 10 * 5 + 10 (bias params per unit) = 60
.
Update. Here is a visual illustration of the example above:
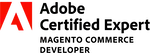